- Across the globe, initiatives point in a single direction – the use of AI in any industry or commercial entity will be governed by laws and regulations requiring strict compliance
- Unless companies are in a regulated industry like financial services or healthcare, not much attention is paid to ensuring AI systems operate responsibly
- So, how do you future proof your AI system for success? Here’s a 6-step process to successful AI deployment
According to the OECD AI Policy Observatory (OECD.AI) – there are over 600 AI policy initiatives from 60 countries, territories, and the EU. These policy initiatives span across various industries and focus on government oversight, guidelines, and regulation on the ethical use of AI. The EU leads the way here with their first ever legal framework to address the risks of AI. In March this year, the US financial regulators put out a request for information on how banks use AI. This shows new guidance or regulation will soon make its way to the US financial sector to govern their use of AI. There is hope that a version of the Algorithmic Accountability Act will soon come into existence to govern AI in the US. Across the globe, these initiatives point in a single direction – the use of AI in any industry will be governed by laws and regulations requiring strict compliance.
What Does Compliance Look Like Today?
Unless companies are in a regulated industry like financial services or healthcare, not much attention is paid to ensuring AI operates responsibly. In financial services, for example, existing regulations mandate that non-explainable AI models cannot make it to production. Often, this stops revenue generating models from being released. Teams are therefore incentivized to make such models inherently more explainable. But in multiple other industries, there are no such guidelines. Many AI models in industries like retail have no limiting factors and a lack of transparency could result in biased models. These companies can face consumer backlash and negative press and will have to go back to the drawing board to make AI more responsible and ethical. Why not start the right way? Build responsibly and ensure you’re future-proofing your AI systems for any and all regulations that will govern AI solutions.
Advocating for More Robust Regulations and Guidelines
The EU regulations, though well-intentioned and one of the first of its kind, can do better to ensure that society as a whole benefits from AI.
Expand what a High Risk System is
All models/systems that serve at scale should be considered high-risk, because of the potential amplification of impact.
Think about Responsible AI Holistically
Rather than limiting guidelines to deployed AI systems only, regulations should oversee other sources, like research projects that could potentially become open sourced and end up in the wrong hands. It’s critical to ensure good model governance practices end-to-end for non-deployed models and data, lest they leak and cause privacy issues. Companies should be required to create a system that allows recourse to the affected end-users, without needing legal involvement.
No Caps on Fines
Instill a minimum for regulatory fines, rather than a maximum. Ensure that revenues from harmful AI systems are recoverable and given to affected communities. A formula for fines could be to take into account the number of people harmed and the extent of the harm.
Mandatory Third-party Training and Auditing
Required AI ethics training from certified third parties for anyone involved in AI will ensure cultural aspects are well-formed. Training should also be extended to those impacted by AI systems. Training and auditing of AI systems should be a must-have. These third-party firms should be certified by a government agency. Companies should use auditing firms to train employees involved in AI development and get a certificate of approval for AI models that directly impact human lives.
Track Long-term Impact of AI Systems
AI governance should include tracking of the long-term impact of AI systems. Very often, it’s impossible to gauge beyond the immediate impact of these systems. Putting regulations and policies in place to track how systems perform over time will install a robust feedback loop to current AI development.
Future proofing AI systems to comply with any and all regulation
Any organization or team working on deploying AI solutions today should be responsible for ensuring that these AI systems are trustworthy and robust. Responsible AI development should belong to everyone. It doesn’t sit with just any one team or an individual like the ethics team or the data scientists or the business leaders. What’s the best way to future proof your AI system for success? Here’s a 6-step process to consider:
1. Educate and train anyone involved
Put together a robust training program to educate everyone involved in AI development to think about all potential risks and harms that AI can cause. Topics can include bias, fairness, ethics, discrimination, etc. as well as more fundamental topics to address foundational aspects like which use cases need AI systems or whether human decisions might be better suited.
2. Create centralized management units
A centralized unit that manages all AI projects is critical to success. If AI projects are scattered across the organization, it will result in chaos – varied tools, guidelines, and opinions are a recipe for disaster. Implement a team that maintains a centralized inventory of all models including their risk levels and types of issues they’ve encountered in the past.
3. Include diverse inputs and sources
Build diverse teams to bring varied viewpoints while building AI systems. Ensure input from those with lived experiences in communities these AI systems will impact. Pay attention to data sources and historical inputs. No matter how diverse you might think your data sources are, it’s likely that when in production, they can still encounter data that will throw them off course and cause potentially biased and harmful outcomes.
4. Ensure accountability and transparency for all AI outcomes
Avoid situations where your team has to make a comment to a customer like, “It’s the algorithm. I don’t know why it did this and I can’t do anything to change it.” Ensure that AI outcomes are transparent and understandable by all stakeholders including employees on the frontlines who engage with customers.
5. Ensure a continuous feedback loop
AI deployment can never follow a one-and-done approach. Feedback from AI systems is critical to ensure they perform as expected. Implement a system that can compare model performance across live, in-production models and those under validation. What discrepancies exist? What biases did your teams uncover? How often did you come across the same issue? Does this warrant a retraining of the model? A feedback loop will answer all these questions and more.
6. Always have humans in the loop
No matter what AI can do and how much faster it can do it, there are still instances where human judgment is critical. Don’t build AI systems in a way that humans can’t override decisions. Always have a fallback in place to ensure a human can come in, get an explanation for an AI outcome, understand why it did what it did, and if it looks incorrect or unfair, then allow the human to change the outcome right away and alert the AI model’s creators to this potential harm.
Authors:
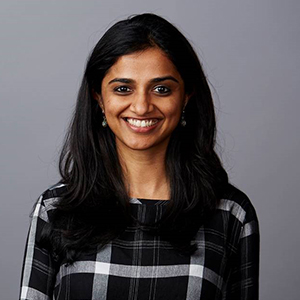
Anusha Sethuraman is a technology product marketing executive with over 12 years of experience across various startups and big-tech companies like New Relic, Xamarin, and Microsoft. She’s taken multiple new B2B products to market successfully with a focus on storytelling and thought leadership. She’s passionate about AI ethics and building AI responsibly, and works with organizations like ForHumanity and Women in AI Ethics to help build better AI auditing systems. She’s currently at Fiddler AI, a Model Performance Management and Explainable AI startup, as VP of Marketing.
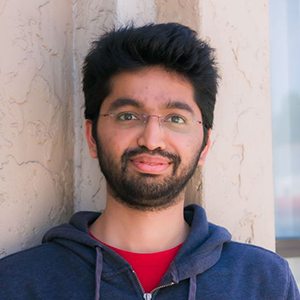
Aalok Shanbhag is a data scientist at Fiddler Labs, where he works on explainable AI, fairness and algorithms for ML model monitoring. He did his Masters in Analytics at Georgia Tech and has an Int. M. Tech in Geophysical Technology from IIT Roorkee.