Researchers at Harvard’s Berkman Klein Center have singled out 32 sets of principles for the responsible use of AI, which they have mapped to eight common themes. The work of applying such principles to the day-to-day world of engineers, practitioners, and, perhaps most importantly, often unsuspecting “passive” subjects affected by the use of AI has begun. The Athens Roundtable, for example, has placed particular emphasis on this question. This post considers the feasibility of ensuring that a society’s core values are operationalized in a government’s use of AI; it does so through the lens of an especially challenging value: fairness.
Fairness as a core value
Fairness, it has been observed, is a pervasive value, one with which we engage in nearly all circumstances of life. Protecting fairness is regularly identified as a core function of government. Fairness in legal proceedings, for example, is an essential element of the rule of law and a basic human right. Guaranteeing fairness in commercial transactions – not in outcome but in procedure – is now an established role for government. Ensuring fairness in land sales between Māori and European settlers was an ostensible objective of the Treaty of Waitangi. Maintaining fairness in access to government services, and to the democratic process itself, is essential to the legitimacy of democratic governments.
The Challenge of Operationalizing Fairness
If fairness is a core value, it must be included as a component of “responsible use” of AI. Doing so, however, is challenged by the lack of a single actionable definition of fairness. Absent such a definition, it is difficult to operationalize the concept via normative instruments.
The definitional difficulties occur at the point of applying the concept; there we encounter questions that admit of no universally applicable answer.
- What unit are we to use in assessing fairness? The individual or the group? If the group, which groups and how defined?
- At what location are we to assess fairness? At the point of opportunity/access? Procedure? Outcome?
- What contextual factors are to be taken into account?
- How do other core values interact with our assessments of fairness?
- How do we account for variation in standards of fairness over time and across cultures? How does the Māori concept of tika coincide (or not) with contemporary English usage of fairness?
The definitional challenges become more acute when we seek quantitative criteria. The community of researchers focused on ensuring fairness in the use of advanced technologies has not reached consensus on metrics. The problem is not a lack of criteria: several have been proposed. The problem is prioritization: it has been repeatedly shown that simultaneously satisfying even three basic criteria for fairness is an impossibility in any real-world scenario. If we have to choose among mutually incompatible criteria, we are back to the challenges faced with natural language definitions of fairness: we have questions the answers to which are highly circumstance-dependent and admit of no universally “correct” answer.
What is to be Done?
What then are policymakers to do? To begin with, let’s note what policymakers should not do. First, they should not rush to simple measures of fairness; while convenient, simple metrics inevitably miss aspects of fairness and thus lack robustness in real-world application, potentially even serving to further unfairness. Second, policymakers should not give up. The mutual incompatibility of fairness metrics “rather than reveal that there are no right answers … show that there are no easy answers.”
What policymakers should do is recognize that the challenges we encounter in defining fairness are challenges we encounter with any core value. Privacy, justice, democracy, the “good” in general are all many-sided, context-dependent, never perfectly realized aspirational values and so are hard to pin down with precise, universally applicable, definitions. This is the challenge Plato brought to the fore with his representations of Socrates pestering his fellow Athenians about their definitions of core values. From this perspective, one could argue that the challenge with fairness is not that it is a vague concept simply in need of better definition; rather, it, like other core values, is an essentially contested concept, one of such internal complexity, circumstance dependency, and moral salience that, by its nature, it invites unresolvable disputes about its proper application. Fairness’s resistance to definition, far from being a flaw, is in fact what gives it its persistent power, as on-going dialogue about its meaning and implications revises and renews it as a core animating value of democratic society.
From Plato to Practitioners
All well and good, a policymaker might say, but I still need a path from this philosophical discussion to something practical. How does this richer understanding of fairness help me develop viable instruments for protecting fairness in the government’s use of AI?
What is needed is a process for the development of normative instruments that is anchored in careful analysis of core values, such as fairness, and the contexts in which those values may be implicated over the lifecycle of an AI-enabled system. Several initiatives seek to help policymakers navigate the path from values to practically viable instruments. The IEEE’s ECPAIS is one; the PHRaE framework, developed by New Zealand’s Ministry for Social Development, is another. One that offers a particularly transparent path from values to instruments is that developed by the Law Committee of the IEEE’s Global Initiative. This approach, represented below, starts from core values and proceeds, step-by-step, to progressively more concrete instantiations of those values until we arrive at truly actionable norms against which real-world practice can be assessed.
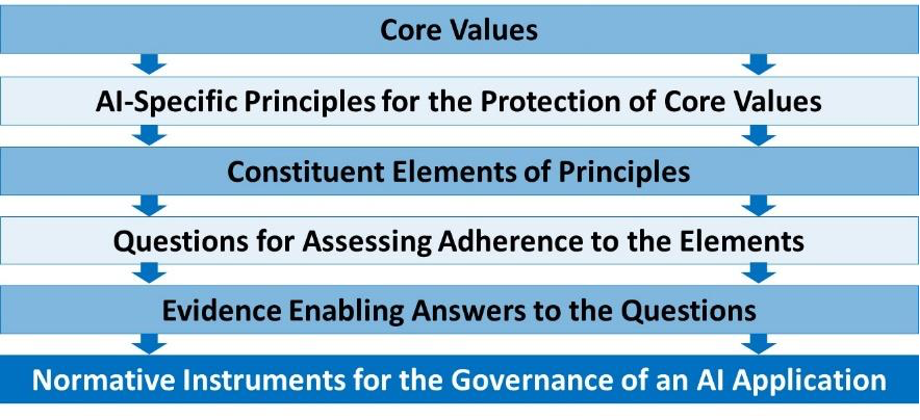
It is not infeasible to operationalize core values, such as fairness, in the use of AI. Doing so, however, starts from an accurate recognition of the nature of the challenge and then, drawing upon a broad base of support, conducting a transparent exploration of the many contexts in which those values will be implicated over the lifecycle of an AI application. It is not easy work, to be sure, but the reward is no less than the realization of robust, practically viable, instruments for the protection of values at the core of a healthy democratic order.